class: title-slide # Chief Analyst Interview <br><br> ### Examples of previous analytical projects <br><br><br><br><br> ### <img src="./assets/img/crop.png" alt="Mainard icon" height="40px" /> Dr Chris Mainey <svg viewBox="0 0 512 512" style="height:1em;position:relative;display:inline-block;top:.1em;fill:#005EB8;" xmlns="http://www.w3.org/2000/svg"> <path d="M459.37 151.716c.325 4.548.325 9.097.325 13.645 0 138.72-105.583 298.558-298.558 298.558-59.452 0-114.68-17.219-161.137-47.106 8.447.974 16.568 1.299 25.34 1.299 49.055 0 94.213-16.568 130.274-44.832-46.132-.975-84.792-31.188-98.112-72.772 6.498.974 12.995 1.624 19.818 1.624 9.421 0 18.843-1.3 27.614-3.573-48.081-9.747-84.143-51.98-84.143-102.985v-1.299c13.969 7.797 30.214 12.67 47.431 13.319-28.264-18.843-46.781-51.005-46.781-87.391 0-19.492 5.197-37.36 14.294-52.954 51.655 63.675 129.3 105.258 216.365 109.807-1.624-7.797-2.599-15.918-2.599-24.04 0-57.828 46.782-104.934 104.934-104.934 30.213 0 57.502 12.67 76.67 33.137 23.715-4.548 46.456-13.32 66.599-25.34-7.798 24.366-24.366 44.833-46.132 57.827 21.117-2.273 41.584-8.122 60.426-16.243-14.292 20.791-32.161 39.308-52.628 54.253z"></path></svg> [@chrismainey](https://twitter.com/chrismainey) <svg viewBox="0 0 496 512" style="height:1em;position:relative;display:inline-block;top:.1em;fill:#005EB8;" xmlns="http://www.w3.org/2000/svg"> <path d="M165.9 397.4c0 2-2.3 3.6-5.2 3.6-3.3.3-5.6-1.3-5.6-3.6 0-2 2.3-3.6 5.2-3.6 3-.3 5.6 1.3 5.6 3.6zm-31.1-4.5c-.7 2 1.3 4.3 4.3 4.9 2.6 1 5.6 0 6.2-2s-1.3-4.3-4.3-5.2c-2.6-.7-5.5.3-6.2 2.3zm44.2-1.7c-2.9.7-4.9 2.6-4.6 4.9.3 2 2.9 3.3 5.9 2.6 2.9-.7 4.9-2.6 4.6-4.6-.3-1.9-3-3.2-5.9-2.9zM244.8 8C106.1 8 0 113.3 0 252c0 110.9 69.8 205.8 169.5 239.2 12.8 2.3 17.3-5.6 17.3-12.1 0-6.2-.3-40.4-.3-61.4 0 0-70 15-84.7-29.8 0 0-11.4-29.1-27.8-36.6 0 0-22.9-15.7 1.6-15.4 0 0 24.9 2 38.6 25.8 21.9 38.6 58.6 27.5 72.9 20.9 2.3-16 8.8-27.1 16-33.7-55.9-6.2-112.3-14.3-112.3-110.5 0-27.5 7.6-41.3 23.6-58.9-2.6-6.5-11.1-33.3 2.6-67.9 20.9-6.5 69 27 69 27 20-5.6 41.5-8.5 62.8-8.5s42.8 2.9 62.8 8.5c0 0 48.1-33.6 69-27 13.7 34.7 5.2 61.4 2.6 67.9 16 17.7 25.8 31.5 25.8 58.9 0 96.5-58.9 104.2-114.8 110.5 9.2 7.9 17 22.9 17 46.4 0 33.7-.3 75.4-.3 83.6 0 6.5 4.6 14.4 17.3 12.1C428.2 457.8 496 362.9 496 252 496 113.3 383.5 8 244.8 8zM97.2 352.9c-1.3 1-1 3.3.7 5.2 1.6 1.6 3.9 2.3 5.2 1 1.3-1 1-3.3-.7-5.2-1.6-1.6-3.9-2.3-5.2-1zm-10.8-8.1c-.7 1.3.3 2.9 2.3 3.9 1.6 1 3.6.7 4.3-.7.7-1.3-.3-2.9-2.3-3.9-2-.6-3.6-.3-4.3.7zm32.4 35.6c-1.6 1.3-1 4.3 1.3 6.2 2.3 2.3 5.2 2.6 6.5 1 1.3-1.3.7-4.3-1.3-6.2-2.2-2.3-5.2-2.6-6.5-1zm-11.4-14.7c-1.6 1-1.6 3.6 0 5.9 1.6 2.3 4.3 3.3 5.6 2.3 1.6-1.3 1.6-3.9 0-6.2-1.4-2.3-4-3.3-5.6-2z"></path></svg> [chrismainey](https://github.com/chrismainey) <svg viewBox="0 0 448 512" style="height:1em;position:relative;display:inline-block;top:.1em;fill:#005EB8;" xmlns="http://www.w3.org/2000/svg"> <path d="M416 32H31.9C14.3 32 0 46.5 0 64.3v383.4C0 465.5 14.3 480 31.9 480H416c17.6 0 32-14.5 32-32.3V64.3c0-17.8-14.4-32.3-32-32.3zM135.4 416H69V202.2h66.5V416zm-33.2-243c-21.3 0-38.5-17.3-38.5-38.5S80.9 96 102.2 96c21.2 0 38.5 17.3 38.5 38.5 0 21.3-17.2 38.5-38.5 38.5zm282.1 243h-66.4V312c0-24.8-.5-56.7-34.5-56.7-34.6 0-39.9 27-39.9 54.9V416h-66.4V202.2h63.7v29.2h.9c8.9-16.8 30.6-34.5 62.9-34.5 67.2 0 79.7 44.3 79.7 101.9V416z"></path></svg> [chrismainey](https://www.linkedin.com/in/chrismainey/) <svg viewBox="0 0 512 512" style="height:1em;position:relative;display:inline-block;top:.1em;fill:#005EB8;" xmlns="http://www.w3.org/2000/svg"> <path d="M294.75 188.19h-45.92V342h47.47c67.62 0 83.12-51.34 83.12-76.91 0-41.64-26.54-76.9-84.67-76.9zM256 8C119 8 8 119 8 256s111 248 248 248 248-111 248-248S393 8 256 8zm-80.79 360.76h-29.84v-207.5h29.84zm-14.92-231.14a19.57 19.57 0 1 1 19.57-19.57 19.64 19.64 0 0 1-19.57 19.57zM300 369h-81V161.26h80.6c76.73 0 110.44 54.83 110.44 103.85C410 318.39 368.38 369 300 369z"></path></svg> [0000-0002-3018-6171](https://orcid.org/0000-0002-3018-6171) <svg viewBox="0 0 496 512" style="height:1em;position:relative;display:inline-block;top:.1em;fill:#005EB8;" xmlns="http://www.w3.org/2000/svg"> <path d="M336.5 160C322 70.7 287.8 8 248 8s-74 62.7-88.5 152h177zM152 256c0 22.2 1.2 43.5 3.3 64h185.3c2.1-20.5 3.3-41.8 3.3-64s-1.2-43.5-3.3-64H155.3c-2.1 20.5-3.3 41.8-3.3 64zm324.7-96c-28.6-67.9-86.5-120.4-158-141.6 24.4 33.8 41.2 84.7 50 141.6h108zM177.2 18.4C105.8 39.6 47.8 92.1 19.3 160h108c8.7-56.9 25.5-107.8 49.9-141.6zM487.4 192H372.7c2.1 21 3.3 42.5 3.3 64s-1.2 43-3.3 64h114.6c5.5-20.5 8.6-41.8 8.6-64s-3.1-43.5-8.5-64zM120 256c0-21.5 1.2-43 3.3-64H8.6C3.2 212.5 0 233.8 0 256s3.2 43.5 8.6 64h114.6c-2-21-3.2-42.5-3.2-64zm39.5 96c14.5 89.3 48.7 152 88.5 152s74-62.7 88.5-152h-177zm159.3 141.6c71.4-21.2 129.4-73.7 158-141.6h-108c-8.8 56.9-25.6 107.8-50 141.6zM19.3 352c28.6 67.9 86.5 120.4 158 141.6-24.4-33.8-41.2-84.7-50-141.6h-108z"></path></svg> [www.mainard.co.uk](https://www.mainard.co.uk) .footnote[Presentation and code available: **https://github.com/chrismainey/chief_analyst_presentation**] .art_cap[R generative art - inspired by Antonio Sánchez Chinchón - @aschinchon] ??? __End 30 seconds__! Say hi - introduce myself I'm Patient Safety Lead - System Analysis and Delivery with NHS England and Improvement. I'm an experienced analyst, data scientist and statistician, used to leading NHS data teams. I'm a senior fellow in the NHS-R community, I contribute to the wider analyst community, and keen to help the profession move forward. Thanks for the opportunity to and interview today. As the brief was to present two projects that demonstrate my data science expertise. I'm committed to using modern techniques, and embracing open source approaches. In part to demonstrate this, the presentation is written in Rmarkdown, wit visualisation and code on GitHub. --- # Two examples of my work: <br><br> ### 1. Developing analytical methods for NHS incident reporting data <br><br> ### 2. Re-imagining standardised mortality ratio (SMR) surveillance in HED ??? __End: 1:00__ I'm aiming to illustrate to problems that I was tackling, give concise summaries of the approaches, highlighting the methods and tool sets used. --- class: inverse center middle # Developing analytical methods for NHS incident reporting data --- ## Analysing Incident Data + My PhD Project: <a name=cite-maineyStatisticalMethodsNHS2020></a>([Mainey, 2020](https://discovery.ucl.ac.uk/id/eprint/10094736/)) thesis available [here](https://discovery.ucl.ac.uk/id/eprint/10094736/) + Patient Safety movement in England has encourage national incident reporting (NRLS) + Routed in visible failures in care such as Mid Staffs and Bristol + Harvard Medical Practice study <a name=cite-leapeNatureAdverseEvents1991></a>([Leape Brennan, et al., 1991](https://doi.org/10/fhvzbs)), 'Too Err is Human' <a name=cite-kohnErrHumanBuilding2000></a>([Kohn Corrigan, et al., 2000](#bib-kohnErrHumanBuilding2000)), 'An organisation with a memory' <a name=cite-donaldsonOrganisationMemory2002></a>([Donaldson, 2002](https://doi.org/10/gfz5w4)) ??? Patient safety movement is reasonably new, established in the 80s through the like of Harvard medical practice study, and the reading, or clinical audit of patient notes and reflections on harm. Often 'imported' from high-risk industries, and incident reporting was ported over, but fairly different in healthcare. + National Reporting and Learning System (NRLS) is the national repository for incident data ### Commonly, with a national dataset there is a desire to use it for benchmarking __End 2:30__ + Desire to compare organisations, and often to 'point the finger', but this is actually at odds with the Patient Safety discipline - about encouraging reporting to learn. + Still desire to understand reporting patterns, but clinical review is main way to use signal. -- + Desire to compare behaviour across organisations safety culture + Little use in most quantitative measures of incident reporting + Change over time? -- + Main signal is free-text: + Clinical review + NLP / text mining -- ### HED wanted an NRLS 'module' for comparing trusts / survellieance ??? ### My project was to examine statistical modelling approaches for these data. --- ## Project overview .left-column[ + __"Ground-clearing" exercise - Literature review__ + Strengthened view on free-text signal + Data completeness a huge issue + Little-to-no useful parameters for modelling + No obvious orthogonal outcome + Reporting is highly organisation-specific {{content}} ] ??? __End 3:30__ To quote Andi when he presented on last week's 'huddle', 'it doesn't get more exciting than a systematic literature search,' but this was the place to start. Deeply challenging review, as it was an ill-defined question: 'how has NRLS been used for analysis?' + 'No orthogonal': Not immediately clear if high reporting is good or bad. It could be that high reporting is a function of a mature organisation that recognises incidents and learns form them, or it could mean that lots of incidents occured. + NLP methods -- + __Only quantitative approach is 'counting'__ + Created a 'panel' dataset - per organisation, per month + Other predictors from HES: APC, OP & AE + Multi-level model assumption due to clustering + Overdispersion modelled various ways -- .right-column[ + __Output approaches for __ + How do we use risk-adjusted output to form indicators? + Visualisation of organisations + Cross-sectional + Longitudinal <br> {{content}} ] -- + __Natural Language Processing__ + Examining how to create a useful dataset + Visualisation of processes + Identify topics in the data + Predictive modelling of harm level from text --- ## Analytical methods (1) ### Data preparation + `SQL Server Database` - ETL + Required manual `SAS` correction for 'delimiting' problems + Panel created using a 'calendar table', join all tables and aggregate per month, per trust. ??? __End 5:00__ + Had to start all the way back with how do we get extracts, as NRLS is not static. Needed system to chose mose recent updated. + Problems with delimiters - required diagnosing and manual SAS process + ETL and warehousing + Extraction into reporting tables as 'counts per day' + HES isn't per day, it's episodes. So per day, is a spell happening? + Investigated different methods of bed-day calculation, depending on which you chose, you can call day cases zero time in hospital. -- ### Predictors / feature engineering + Predictors examined as raw counts, and proportions, as well as 'offset' denominators + Functional forms examined - e.g. percentile representation, multi-collinearity issues, and splines. -- ### Regression-based models: + Poisson regression <a name=cite-poissonRecherchesProbabiliteJugements1837></a>([Poisson, 1837](#bib-poissonRecherchesProbabiliteJugements1837)) (`R`) as basis, in `R` + Mixture/compound distribution models <a name=cite-sellersFlexibleRegressionModel2010></a>([Sellers and Shmueli, 2010](https://doi.org/10/cn6mjv)) --- ## Analytical methods (2) .pull-left[ ___Overdispersion___, where conditional variance is greater than conditional mean, occurs when: 1. Aggregation / Discretization 1. Mis-specified predictors/model 1. Presence of outliers 1. Variation between response probabilities (heterogeneity) {{content}} ] -- __Repeated measures (correlation) __ + Regression assumes all points independent + Sampling from same organisations repeatedly + Clustered: - local means -- .pull-right[ 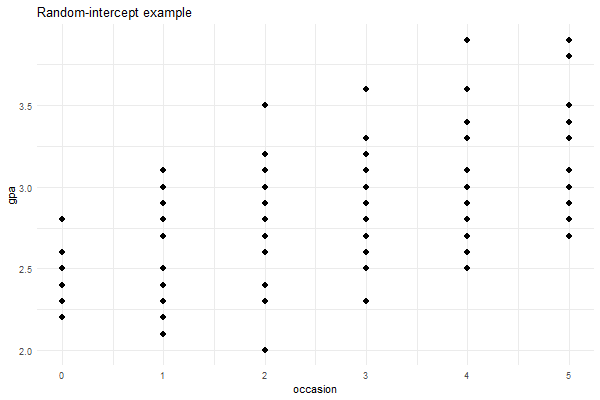 ] ??? __End 6:00__ + The big issue here is overdispersion. For distributions with fixed variance assumption (Poisson and Binomial), there isn't room in the mathematical definition for anything other than perfect. + That is to say that, the model assumes it's a perfect fit, and it's not. Mainly from: --- ## Analytical methods (3) .pull-left[ ###Advances on Poisson regression + Generalized Additive Models (GAM):<a name=cite-woodGeneralizedAdditiveModels2006></a>([Wood, 2006](#bib-woodGeneralizedAdditiveModels2006)) + smoothed functions of predictors + Tree methods: + "Boosting" <a name=cite-friedmanStochasticGradientBoosting2002></a>([Friedman, 2002](https://doi.org/10/fxb956)) + "Bagging" & Random Forests <a name=cite-breimanBaggingPredictors1996></a><a name=cite-breimanRandomForests2001></a>([Breiman, 1996](https://doi.org/10/fm3fwj); [Breiman, 2001](https://doi.org/10/d8zjwq)) + Neural Networks <a name=cite-hastieElementsStatisticalLearning2009></a>([Hastie, Tibshirani, and Friedman, 2009](#bib-hastieElementsStatisticalLearning2009)) ] -- .pull-right[ ### Use of models + Indirectly standardised ratio + Overdispersion adjusted z-scores <a name=cite-spiegelhalterHandlingOverdispersionPerformance2005></a>([Spiegelhalter, 2005b](https://doi.org/10/cdmxpn)) + Funnel plots <a name=cite-spiegelhalterFunnelPlotsComparing2005></a>([Spiegelhalter, 2005a](https://doi.org/10/fq7z8t)) + Control charting <a name=cite-woodallUseControlCharts2006></a>([Woodall, 2006](https://doi.org/10/gjzktt)) ] ??? _Ends 7:30__ + GAMS - probably one of my favourite methods as they are good at characterising the relations and reducing the noise (bias - variance trade-off) + Tree are a completely different type of regression model that involves recursively splitting data at points that explain the most variance. Give similar results to MLE, becuse both describing the data, but some differences. Need to control for bias and over-fitting, so bootstrapping ('bagging') and boosting (iteratively refitting weighted on error). + Neural networks, at simplest are cascade of rsimple models, stacking in parallel with 'layers' in sequence. So called 'deep learning' indicates networks with more layers. Fitted a simple feed-forward network. Little advantage to these on small datasets. --- ## Natural Language Processing (NLP) Key that the free-text description contains real signal, but can't manually read ~500,000 per year .pull-left[ + Quantitative analysis of text + Text processing / preparation `tidytext`, `quanteda` + Visualisation - wordclouds, TF-IDF + Topic Modelling - LDA <a name=cite-bleiLatentDirichletAllocation2003></a>([Blei Ng, et al., 2003](#bib-bleiLatentDirichletAllocation2003)) + Using LDA predictions to predict harm level + __Class-imbalance__ ] .pull-right[ <p style="text-align:center;"> <img src="./assets/img/wordcloud.png" alt="Wordclouds showing stage of NRLS cleaning" width = "400" height="350"> </P> ] ??? 'Proverbial elephant elephant in the room' The key issue here is that there are ~500,000 reports per year. National Clinical review team of 3 -5 depending on project, so they focus on severe harm and death. With routing research and other work, maybe a further 2% are systematically reviewed. This means 98% are not reviewed and, to my mind, 'begging' for some methods of finding a signal. This is where text methods come in. Class imbalance is the major issue here: that is where there are different size classes (or groups) to predict. As said before Severe and and Death account for >1%. Naturally poorer at predicting these, but interesting finding what that Random Forest methods performed better than others against class imbalance ~83% accuracy, and Naive Bayes performed well for minority classes by not majority. --- ## Outputs + HED interactive 'module' allowing - NHSEI prevented launch of this, but techniques used to improve several other parts of HED. + Recommendations for modelling shared with organisations: + NHSD now investigating some of methods for SHMI. + NHSEI simple method of 'under-reporting' greatly advanced by these models. + NLP methods have been recognised as way forward to make better use of data. + In moving to NHSEI patient safety team, I am starting to implement these methods for national team. + Creation of `FunnelPlotR` R package, available on CRAN and donated to NHS-R community. ??? __End 8:00__ --- class: inverse center middle # Re-imagining standardised mortality ratio (SMR) surveillance in HED --- ## Re-imagining SMR surveillance + When comparing hospital mortality, it is common to adjust for "case-mix" to reduce confounding. + Organisation conflate 'excess' predicted deaths with 'preventable' deaths, and much academic debate .pull-left[ + Commonly measures are: + Summary Hospital-level Mortality Indicator (SHMI) <a name=cite-campbellDevelopingSummaryHospital2012></a>([Campbell Jacques, et al., 2012](https://doi.org/10/gb3r9t)) + Dr Foster Hospital Standardised Mortality Ratio (HSMR) <a name=cite-jarmanExplainingDifferencesEnglish1999></a>([Jarman Gault, et al., 1999](https://doi.org/10/fkkfm9)) + Commonly make the cross-sectional comparisons with Funnel Plot + __Unanswered question:__ + 'Is trust "X" getting worse'? ] .pull-right[ <a href="https://github.com/nhs-r-community/FunnelPlotR" target="_blank"><img src="chief_analyst_interview_files/figure-html/funnel-1.png" title="Example funnel plot" alt="Example funnel plot" width="600" height="300" /></a> ] ??? First point: reduces confounding, but sometimes increases bias and is at the expense of complexity. --- ## Monitoring SMRs over time .pull-left[ ### Risk-adjusted cusum charts + Two differing approaches: + CQC outliers programme + Dr Foster's cususm alerts + Organisations don't understand as 'diagnostic test' {{content}} ] .pull-right[ <p style="text-align:center;"> <img src="./assets/img/agg_cusum.gif" alt="Aggregate cusum chart" width = "400" height="200"> <img src="./assets/img/person_cusum.gif" alt="Person-level cusum chart" width = "400" height="200"> </p> ] ??? For methods with more of a time dimension, one might consider forecasting of survival methods, in general. Dr Foster Intelligence created an industry or 'alerting' trusts to risks - based on the methods they CQC The diagnostic test, the same in any machine learning prediction model, is essential. In epidemiology and machine learning we might construct a 2x2 table, or confusion matrix, with our predictions v.s. truth. Assess quality of model, but there are always some false positive and negatives. There is a trade-off between 'sensitivity' and 'specificity': You can catch more with a lower threshold, but you'll get more false positives. Extreme of this is to treat every data point as positive. This has resource implications - requiring clinical review and often highlighting data quality or statistical issues. -- + Unclear why there were differences: + Aggregation + Trigger limit + 'Window' size + __Both claimed false discovery rate (FDR) of 0.1%__ --- ### Developing methods .pull-left[ ### CQC + Data are transformed to z-scores + Global trigger (5.48) - + Can convert average run-length to FDR, and set threshold <a name=cite-griggNullSteadystateDistribution2008></a><a name=cite-carequalitycommissioncqcNHSAcuteHospitals2014></a>([Grigg and Spiegelhalter, 2008](https://doi.org/10/bgvkdx); [Care Quality Commission, 2014](#bib-carequalitycommissioncqcNHSAcuteHospitals2014)) + Applicable to other indicators and groupings, subject to transformation + Tested in R, translated in `SQL` procedures for calculation against all trusts and diagnosis groups ] -- .pull-right[ ### DFI + Binomial assumption and threshold set through simulation of average run-length to false positives. <a name=cite-bottlePredictingFalseAlarm2011></a>([Bottle and Aylin, 2011](https://doi.org/10/cbr4rq)) + Unique to each trust / group / reporting period. + Intractable to calculate each month, and authors fitted a set of descriptive equations. + Wrote program to solve equations for each group with non-linear optimiser (`R`) + Translated into similar `SQL` process, with call to `R` ] --- ## Delivery and presentation ### Technical + Takes too long! + Iterative ~ 160 organisations \* 260 diagnosis groups \* 2 methods. + Tested multiple languages and data structures, designs + SQL 'quirky update' - best performance -- ### Display + Important to place charts together to see trigger times + Different aggregations + Balance of detail and simplicity + Linked to email alerts and dashboard summary + Patient details - audit -- __Educate trusts on false-positive argument and build confidence, and skills to investigate.__ --- class: references ### References (1) <a name=bib-bleiLatentDirichletAllocation2003></a>[Blei, D. M., A. Y. Ng, and M. I. Jordan](#cite-bleiLatentDirichletAllocation2003) (2003). "Latent dirichlet allocation". In: _J. Mach. Learn. Res._ 3, pp. 993-1022. ISSN: 1532-4435. <a name=bib-bottlePredictingFalseAlarm2011></a>[Bottle, A. and P. Aylin](#cite-bottlePredictingFalseAlarm2011) (2011). "Predicting the false alarm rate in multi-institution mortality monitoring". In: _The Journal of the Operational Research Society_ 62.9, pp. 1711-1718. ISSN: 01605682, 14769360. DOI: [10/cbr4rq](https://doi.org/10%2Fcbr4rq). <a name=bib-breimanBaggingPredictors1996></a>[Breiman, L.](#cite-breimanBaggingPredictors1996) (1996). "Bagging predictors". In: _Machine Learning_ 24.2, pp. 123-140. ISSN: 1573-0565. DOI: [10/fm3fwj](https://doi.org/10%2Ffm3fwj). <a name=bib-breimanRandomForests2001></a>[Breiman, L.](#cite-breimanRandomForests2001) (2001). "Random Forests". In: _Machine Learning_ 45.1, pp. 5-32. ISSN: 08856125. DOI: [10/d8zjwq](https://doi.org/10%2Fd8zjwq). <a name=bib-campbellDevelopingSummaryHospital2012></a>[Campbell, M. J., R. M. Jacques, J. Fotheringham, et al.](#cite-campbellDevelopingSummaryHospital2012) (2012). "Developing a summary hospital mortality index: retrospective analysis in English hospitals over five years". In: _BMJ_ 344, p. e1001. DOI: [10/gb3r9t](https://doi.org/10%2Fgb3r9t). <a name=bib-carequalitycommissioncqcNHSAcuteHospitals2014></a>[Care Quality Commission](#cite-carequalitycommissioncqcNHSAcuteHospitals2014) (2014). _NHS acute hospitals: Statistical Methodology_. Care Quality Commission (CQC). <a name=bib-donaldsonOrganisationMemory2002></a>[Donaldson, L.](#cite-donaldsonOrganisationMemory2002) (2002). "An organisation with a memory". In: _Clinical Medicine (London, England)_ 2.5, pp. 452-7. ISSN: 1470-2118 (Print) 1470-2118. DOI: [10/gfz5w4](https://doi.org/10%2Fgfz5w4). <a name=bib-friedmanStochasticGradientBoosting2002></a>[Friedman, J. H.](#cite-friedmanStochasticGradientBoosting2002) (2002). "Stochastic Gradient Boosting". In: _Computational Statistics & Data Analysis_ 38, pp. 367-378. DOI: [10/fxb956](https://doi.org/10%2Ffxb956). <a name=bib-griggNullSteadystateDistribution2008></a>[Grigg, O. and D. Spiegelhalter](#cite-griggNullSteadystateDistribution2008) (2008). "The null steady-state distribution of the CUSUM statistic". In: _Technometrics : a journal of statistics for the physical, chemical, and engineering sciences_. DOI: [10/bgvkdx](https://doi.org/10%2Fbgvkdx). <a name=bib-hastieElementsStatisticalLearning2009></a>[Hastie, T., R. Tibshirani, and J. Friedman](#cite-hastieElementsStatisticalLearning2009) (2009). _The Elements of Statistical Learning : Data Mining, Inference, and Prediction_. 2nd ed. Springer Series in Statistics. New York, NETHERLANDS: Springer. ISBN: 978-0-387-84858-7. <a name=bib-jarmanExplainingDifferencesEnglish1999></a>[Jarman, B., S. Gault, B. Alves, et al.](#cite-jarmanExplainingDifferencesEnglish1999) (1999). "Explaining differences in English hospital death rates using routinely collected data". In: _BMJ_ 318.7197, pp. 1515-1520. ISSN: 0959-8138 1468-5833. DOI: [10/fkkfm9](https://doi.org/10%2Ffkkfm9). <a name=bib-kohnErrHumanBuilding2000></a>[Kohn, L. T., J. M. Corrigan, and M. S. Donaldson](#cite-kohnErrHumanBuilding2000) (2000). "To Err is Human: Building a Safer Health System". In: _To Err is Human: Building a Safer Health System_. Ed. by L. T. Kohn, J. M. Corrigan and M. S. Donaldson. Washington (DC). <a name=bib-leapeNatureAdverseEvents1991></a>[Leape, L. L., T. A. Brennan, N. Laird, et al.](#cite-leapeNatureAdverseEvents1991) (1991). "The Nature of Adverse Events in Hospitalized Patients". In: _New England Journal of Medicine_ 324.6, pp. 377-384. DOI: [10/fhvzbs](https://doi.org/10%2Ffhvzbs). <a name=bib-maineyStatisticalMethodsNHS2020></a>[Mainey, C.](#cite-maineyStatisticalMethodsNHS2020) (2020). "Statistical methods for NHS incident reporting data". London. URL: [https://discovery.ucl.ac.uk/id/eprint/10094736/](https://discovery.ucl.ac.uk/id/eprint/10094736/). --- class: references ### References (2) <a name=bib-poissonRecherchesProbabiliteJugements1837></a>[Poisson, S. D.](#cite-poissonRecherchesProbabiliteJugements1837) (1837). _Recherches sur la probabilité des jugements en matiére criminelle et en matière civile: précédées des règles générales du calcul des probabilités_. Bachelier. <a name=bib-sellersFlexibleRegressionModel2010></a>[Sellers, K. F. and G. Shmueli](#cite-sellersFlexibleRegressionModel2010) (2010). "A flexible regression model for count data". In: _Annals of Applied Statistics_ 4.2, pp. 943-961. ISSN: 1932-6157. DOI: [10/cn6mjv](https://doi.org/10%2Fcn6mjv). <a name=bib-spiegelhalterFunnelPlotsComparing2005></a>[Spiegelhalter, D. J.](#cite-spiegelhalterFunnelPlotsComparing2005) (2005a). "Funnel plots for comparing institutional performance". In: _Statistics in Medicine_ 24.8, pp. 1185-202. ISSN: 0277-6715 (Print) 0277-6715 (Linking). DOI: [10/fq7z8t](https://doi.org/10%2Ffq7z8t). <a name=bib-spiegelhalterHandlingOverdispersionPerformance2005></a>[Spiegelhalter, D. J.](#cite-spiegelhalterHandlingOverdispersionPerformance2005) (2005b). "Handling over-dispersion of performance indicators". In: _Quality and Safety in Health Care_ 14.5, pp. 347-351. DOI: [10/cdmxpn](https://doi.org/10%2Fcdmxpn). <a name=bib-woodGeneralizedAdditiveModels2006></a>[Wood, S. N.](#cite-woodGeneralizedAdditiveModels2006) (2006). _Generalized Additive Models: An Introduction with R_. Taylor & Francis. ISBN: 978-1-58488-474-3. <a name=bib-woodallUseControlCharts2006></a>[Woodall, W. H.](#cite-woodallUseControlCharts2006) (2006). "The Use of Control Charts in Health-Care and Public-Health Surveillance". In: _Journal of Quality Technology_ 38.2, pp. 89-104. ISSN: 0022-4065. DOI: [10/gjzktt](https://doi.org/10%2Fgjzktt).